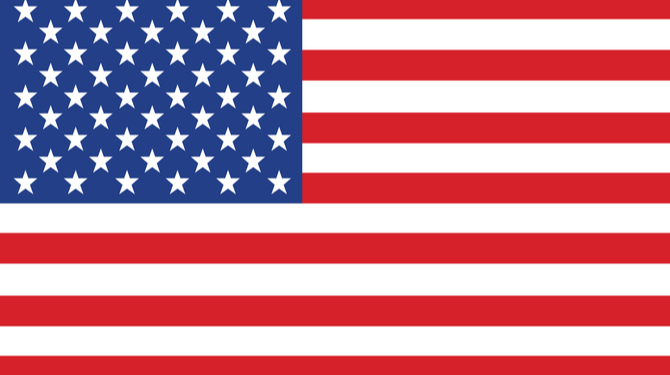
United States
Artificial Intelligence
This is the first edition of the Law Over Borders Artificial Intelligence guide. The second edition is now available for viewing and purchase: Artificial Intelligence
Introduction
The term “Artificial Intelligence” is very broad, including many different areas that mimic human intelligence. AI is a fundamental technology used by virtually all other industries, and is not a separate industry by itself. The analysis below falls into three broad categories:
- intellectual property protection for AI and the data used by AI;
- legal regimes to protect against AI abuse (e.g., privacy); and
- how to handle AI inventors and authors.
In the United States, intellectual property protection is fairly stable, even if not completely understood. The legal regimes to protect against abuse are being developed and implemented as people discover the extent of what AI can do. And the question of how to handle AI inventors/authors is in the early investigative stage.
1 . Constitutional law and fundamental human rights
The United States Constitution and the constitutions of individual states did not foresee the development of AI, so there are no provisions that are specific to AI. However, many of the constitutional provisions can be readily applied in an AI context. This is also true for fundamental human rights.
1.1. Domestic constitutional provisions
Multiple portions of the U.S. Constitution protect the rights of people, including the Fourth Amendment and the Fourteenth Amendment. These two amendments will apply if an AI system is invoked in a way that attempts to abridge the rights of individuals.
The Fourth Amendment limits search and seizure, and requires “probable cause, supported by Oath or affirmation.” Although an AI system could be trained to identify the “probable cause” of illegal activity, U.S. courts would most likely rule that an AI system could not provide an “oath or affirmation,” and therefore would not permit a search or seizure based on AI analysis alone. (Analysis by an AI system could supplement other evidence that shows probable cause.) Because an AI system to identify probable cause does not exist yet, no court has ruled on this question.
The “Due Process” clause of the Fourteenth Amendment has been construed broadly over the past 150 years, and would likely preclude deprivation of “life, liberty, or property” based solely on an AI system. At present, the processing of an AI system is a “black box,” so the Fourteenth Amendment should not permit conviction based on an unexplained “conclusion” of an AI system (even if the conclusion is correct).
1.2. Human rights decisions and conventions
Unlike other countries, a court in the United States is unlikely to reason about human rights without citation to the Constitution or statutory law. The human rights most relevant to AI are in the U.S. Constitution, as discussed in Section 1.1 Domestic constitutional provisions, above.
2 . Intellectual property
There are at least two key issues at the intersection of AI and intellectual property. The first key issue involves protection of inventions that utilize AI. For this issue, the sub-questions are:
- is the invention patentable;
- is the invention protectable as a trade secret; and
- if both patent and trade secret protection are possible, what criteria can be applied to make the choice.
The second key issue involves protection of inventions or original works created by an AI system. The simple answer here is that inventions and original works created solely by an AI system are not protectable with patents or copyrights. This issue can be addressed in some ways, as discussed in Sections 2.2 Copyright and 2.3 Trade secrets/confidentiality.
Because many AI systems are implemented in software, it is also possible to protect the source code under copyright law. However, this protection is relevant only to theft by individuals who have direct access to the source code.
2.1. Patents
Inventions that use AI
There are many inventions that use AI, and the trend is increasing. The AI is usually one aspect of an apparatus or method, and U.S. patent examiners apply the standard rules to the invention as a whole. There are two important caveats:
- machine learning is very well known, so the inventive aspect must be something other than machine learning; and
- the inventive aspect must be claimed in sufficient detail to avoid being rejected as an “abstract idea.”
A large portion of AI falls into the category of “machine learning,” in which the AI system is trained to recognize or classify. But the label “AI” applies to more than machine learning (such as speech generation).
For inventions that utilize machine learning, the invention typically uses “off the shelf” machine learning systems (such as neural networks or decision trees), so the machine learning aspect should be ignored when evaluating patentability. If everything else is known or obvious, the invention is not patentable.
On the other hand, if an AI invention is a new or enhanced AI algorithm, patentability is primarily based on claiming the algorithm properly.
Inventions created by an AI system
Inventions that are created solely by an AI system are not patentable because U.S. patent law currently requires a human inventor. See, for example, Thaler v. Vidal, No. 21-2347 (Fed. Cir. August 5, 2022). Because of this, companies should create development processes that have at least one human inventor. Even if a fully autonomous AI development system is faster and cheaper, it may not be useful if the created inventions cannot be patented.
2.2. Copyright
Some AI systems are currently able to create high-quality art (e.g., visual, musical, or linguistic), but the results cannot be copyrighted because there is no human author/creator. The U.S. case of the “monkey selfie” showed that a non-human cannot own a copyright, and that result would apply to art created by AI systems.
It is an open question if a copyright could be granted for work that was created jointly by a human and an AI system. It is likely that a human artist/creator could file for a copyright as long as the human contribution is a non-trivial part of the “art.”
2.3. Trade secrets/confidentiality
Trade secrets are not subject to patent law, so there is no requirement for a human inventor, no requirement to prove novelty, and no requirement to prove subject matter eligibility (i.e., showing an invention is not an “abstract idea”). Therefore, trade secret protection is an attractive option.
For an invention that uses AI or was developed by AI, the key factors to consider are:
- whether it is possible to keep the invention hidden from those trying to reverse engineer; and
- whether it would be possible to detect infringers.
Trade secret protection is generally a good option when it is possible to keep the invention hidden and/or it would be difficult/impossible to detect patent infringement.
In addition to protection of systems or methods, it is almost always useful to protect relevant data as a trade secret. For example, a system that uses machine learning uses training data, and that training data can be very valuable based on the time and resources needed to collect and classify it. In addition, a trained machine learning model can be kept as a trade secret. The trained model is just a large batch of parameters (e.g., neural network weights), and these parameters are not visible when the model is used.
3 . Data
3.1. Domestic data law treatment
The U.S. does not have a unified law that covers data protection. Instead, there is a mix of laws enacted on both the federal and state levels to protect the personal data of people.
Federal laws tend to focus on specific types of data. For example:
- The Health Insurance Portability and Accountability Act (HIPAA) (29 U.S.C. § 1181 et seq.) sets the standard for protecting sensitive patient data information;
- The Fair Credit Reporting Act (FCRA) (15 U.S.C. § 1681) protects information collected by consumer reporting agencies; and
- The Children’s Online Privacy Protection Rule (COPPA) (15 U.S.C. § 6501 et seq.) prohibits the collection or use of personal information from and about children under the age of 13 on the Internet.
State laws may impose restrictions relating to the collection and use of data, such as biometric data. See below Section 3.4 Biometric data.
3.2. General data protection regulation
See above Section 3.1 Domestic data law treatment.
In the European Union and the European Economic Area, data privacy is regulated by the General Data Protection Regulation (GDPR). GDPR is not applicable to the U.S. In March 2022, the United States and the European Commission committed to a new Trans-Atlantic Data Privacy Framework, which will regulate trans-Atlantic data flows and address the concerns raised by the Court of Justice of the European Union in 2020 when it struck down the Commission’s adequacy decision underlying the EU-U.S. Privacy Shield framework.
3.3. Open data & data sharing
In June 2021, the Biden administration created the National Artificial Intelligence Research Resource (NAIRR) Task Force to address the growing resource divide of AI and to democratize access for AI research and development.
In its May 2022 Interim Report, the Task Force’s recommendations with regards to data include:
- designing a NAIRR resource allocation framework that incentivizes the contribution of high-quality data and metadata to the user community or to the public good;
- establishing an ecosystem around data that can be used for AI and to support data search and discovery;
- facilitating access to three types of government data: statistical data, administrative data, and data generated by federally funded research;
- protecting privacy by following the “Five Safes” framework for safe use (safe projects, safe people, safe data, safe settings, and safe outputs); and
- implementing a tiered access model for confidential or sensitive data to accommodate heterogeneous security needs.
Government agencies often have legal responsibilities that prevent or create duties related to data sharing. For example, the use of Federal statistical data is subject to the Confidential Information Protection and Statistical Efficiency Act. Census data is subject to U.S.C. Title 13, and Federal tax information use is subject to U.S.C. Title 26. These responsibilities will likely remain when sharing data for AI research and development.
3.4. Biometric data: voice data and facial recognition data
The U.S. does not have a federal privacy law. States have the authority to protect the personal data of its citizens. Usage of AI systems is subject to all of the state laws.
Illinois was the first state to enact a law restricting the collection and storage of biometrics. The Illinois Biometric Information Privacy Act (BIPA) requires entities that collect biometric data to follow a number of protocols, including maintaining a written policy about the collection and storage of biometric data and obtaining informed consent from individuals subject to biometric data collection.
Under the Capture or Use of Biometric Identifier (CUBI) Act in Texas, a private entity may not capture a person’s biometric identifier for a commercial purpose unless the entity informs the person prior to capturing the biometric identifier and receives the person’s consent to the collection.
In California, biometric data – including voice data and facial recognition data – is protected information under the California Consumer Privacy Act (CCPA) and the California Privacy Rights Act (CPRA). California also restricts the use of facial recognition technology by law enforcement agencies.
In Virginia, the Virginia Consumer Data Protection Act (VCDPA) requires controllers to obtain consent before processing a consumer’s biometric data.
Washington has a biometric privacy law known as H.B. 1493, to safeguard its residents from organizations or individuals who “enroll” a biometric identifier into a database without providing notice, obtaining consent, or providing a mechanism to prevent the use of biometric data for commercial purposes.
4 . Bias and discrimination
While large data sets can give insight into previously intractable challenges, hidden biases at both the collection and analytics stages of big data’s life cycle can lead to disparate impact.
4.1. Domestic anti-discrimination and equality legislation treatment
A number of federal equal opportunity laws, such as the Equal Credit Opportunity Act (ECOA), Title VII of the Civil Rights Act of 1964, the Americans with Disabilities Act, the Age Discrimination in Employment Act, the Fair Housing Act, and the Genetic Information Non-discrimination Act, prohibit discrimination based on protected characteristics. These laws all apply when using AI.
Of these laws, the Federal Trade Commission (FTC) enforces the ECOA, which prohibits credit discrimination on the basis of race, color, religion, national origin, sex, marital status, age, or because a person receives public assistance.
In its 2016 Big Data Report, the FTC warned companies that big data analytics could result in bias or other harm to consumers. To avoid that outcome, any operator of an algorithm should ask four key questions:
- How representative is your data set? Companies should consider whether their data sets are missing information about certain populations, and take steps to address issues of underrepresentation and overrepresentation.
- Does your data model account for biases? Companies should consider whether biases are being incorporated at both the collection and analytics stages of big data’s life cycle, and develop strategies to overcome them.
- How accurate are your predictions based on big data? Companies should remember that while big data is very good at detecting correlations, it does not explain which correlations are meaningful.
- Does your reliance on big data raise ethical or fairness concerns? Companies should assess the factors that go into an analytics model and balance the predictive value of the model with fairness considerations.
When the FTC evaluates an AI algorithm for illegal discrimination, it looks at whether the model includes ethnically-based inputs, or proxies for inputs. It also reviews the outcomes regardless of the inputs, to determine whether a model appears to have a disparate impact on people in a protected class. If it does, the FTC then reviews the company’s justification for using that model and consider whether a less discriminatory alternative would achieve the same results.
5 . Trade, anti-trust and competition
5.1. AI related anti-competitive behaviour
One question that arises in light of antitrust law is whether companies can be liable when their AI algorithms learn to adopt collusive pricing rules without human intervention or even knowledge.
Price collusion claims can be litigated under either Section 1 of the Sherman Act or Section 5 of the Federal Trade Commission (FTC) Act. Under Section 1 of the Sherman Act, price collusion can be per se illegal. However, the statutory case law requires either direct or circumstantial evidence of an agreement between competitors to fix prices to prove a statutory violation. On the other hand, Section 5 of the FTC Act does not require an explicit showing of an agreement for antitrust claims. Even then, most price-fixing cases tend to be brought under the Sherman Act because the FTC has expressed reluctance to challenge practices on a standalone section 5 basis when the Sherman Act could sufficiently address the uncompetitive practice.
In 2017, then-Federal Trade Commissioner Terrell McSweeny noted that while the use of a pricing algorithm, by itself, does not raise antitrust concerns, the potential that pricing algorithms will facilitate tacit collusion beyond the reach of Section 1 of the Sherman Act is far from fanciful. Consequently, Section 5 of the FTC Act may be the only current tool available to police individual instances of algorithmic collusion.
Another question is whether companies whose AI algorithms learn to adopt collusive pricing rules can be liable under respondeat superior. It is unclear whether AI could be considered an “employee” for such purposes, or considered a conscious decision maker that could “agree” to collude.
5.2. Domestic regulation
In the U.S., antitrust law is a collection of mostly federal statutes. These include the Sherman Act of 1890, the Clayton Act of 1914, and the Federal Trade Commission (FTC) Act of 1914.
6 . Domestic legislative developments
Regulations on the usage of AI in employment
States in the United States have begun regulating the usage of AI in recruiting processes. Illinois, Maryland, and New York City have laws that require notifying applicants when AI will be used so evaluate application materials or interviews, and may require consent of the applicant. See “Artificial Intelligence Video Interview Act,” Public Law 101-0260 (Illinois), “Labor and Employment – Use of Facial Recognition Systems – Prohibition,” 2020 H.B. 1202 (Maryland), and “Automated Employment Decision Tools,” Int. 1894-2020A (New York City).
California has prepared a draft law that goes much further, with the specific goal of preventing the use of AI when it leads to systematic discrimination against protected classes of individuals. See Workplace Technology Accountability Act (California Assembly Bill 1651). Under the proposed law, employers could be subject to liability even when there is no discriminatory intent.
The proposed California law is likely to be enacted in substantially its current form, and many other states are likely to adopt similar policies over time.
Update to patent law to allow AI inventors
Although US patent law does not currently allow AI inventors, the existence and increase in AI inventors will require changes to US patent law. Further, because patent applications filed in the United States are commonly filed in other countries, adapting the laws may require a coordinated effort. The USPTO has sought input regarding AI inventors and the usage of AI more generally, but there are no current legislative proposals.
In the case Thaler v. Vidal, a developer of an AI system called “DABUS” filed a patent application that listed DABUS as the sole inventor. The USPTO rejected the application because there is no human inventor, and the District Court for the Eastern District of Virginia affirmed the rejection. The Federal Circuit affirmed the ruling of the lower court on August 5, 2022. It is up to the US Congress whether to draft new patent laws that allow AI inventors.
7 . Frequently asked questions
1. How can you protect an AI system when you don’t know what the AI is doing?
This question generally occurs when an invention uses machine learning. An inventor identifies the inputs and output, and collects some training data. For example, a system to identify spam email may have a training set of data consisting of 10,000 emails, and each of those emails is classified as spam or not. Based on the training, the system is able to classify new emails as spam or not, but the black box system does not specify how the decision is made.
As described in Section 2.1 Intellectual Property: Patents, patentability is based on the overall process, and the internal workings of the machine learning are not important because they are not part of the patentability analysis. The patentability comes from somewhere else, such as the methodology used to collect the input data, the methodology to process the input data, or the methodology of using the output.
2. For an invention that uses AI, how can I decide whether to seek a patent or keep it as a trade secret?
This is discussed in Section 2.3 Intellectual Property: Trade secrets/confidentiality. For an invention that uses AI, a key question is “what part of the process is inventive?” If the inventive feature is the specific data that provides input to a machine learning system, the data is likely to be visible, so patent protection is usually needed. On the other hand, if the inventive aspect is part of the AI itself (such as a new Natural Language algorithm or Image Processing algorithm), trade secret protection is generally preferable. In short, to make a decision between patent and trade secret protection, (1) identify the inventive feature (which may not be the AI), and then (2) determine whether the inventive feature is visible.
3. Can I actually get a patent for a software system that uses AI?
In 2014, the U.S. Supreme Court issued a decision for Alice v. CLS Bank, which addressed “subject matter eligibility” (whether patent claims are directed to an abstract idea). Despite the fact that the Court just affirmed its own precedent, the Federal Circuit has subsequently issued a variety of opinions that have substantially increased the number of rejections for subject matter eligibility. It is definitely still possible to get software patents, but it is more work to prepare a patent application and good patent claims, and success can depend on the assigned examiner.
Many AI inventions are substantially software applications, so the additional burden applies.
3-6 PQE Corporate M&A Associate
Job location: London
Projects/Energy Associate
Job location: London
3 PQE Banking and Finance Associate, Jersey
Job location: Jersey